Uncategorized
5 tips for getting started with generative AI
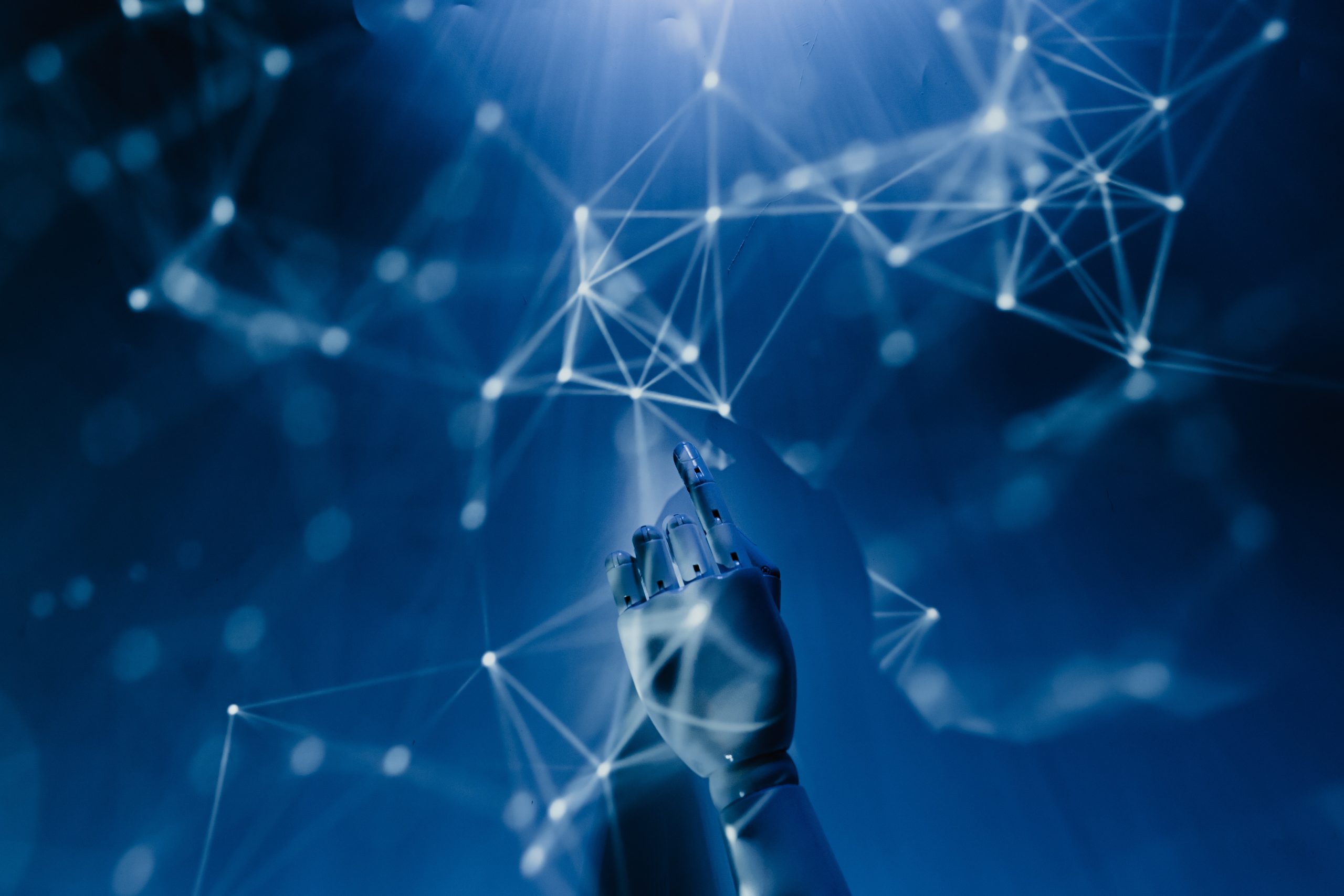
Artificial intelligence has revolutionized many industries, and one of the most fascinating areas of AI is generative AI. By using complex algorithms and machine learning techniques, generative AI can create original content such as images, music, and even entire stories. It has the ability to produce impressive results that seem almost human-like, sparking curiosity and interest in both professionals and enthusiasts alike. If you’re eager to dive into the world of generative AI but don’t know where to start, fear not! In this article, we’ll provide you with five essential tips that will help you begin your journey into this captivating field.
What is generative AI
Generative AI, also known as generative adversarial networks (GANs), is a subset of artificial intelligence that focuses on creating new content rather than simply processing or analyzing existing data. Unlike traditional machine learning algorithms, which rely on large datasets to learn patterns and make predictions, generative AI aims to generate entirely new data based on the patterns it has learned. Think of it as an AI-powered creative engine that can produce realistic images, videos, music, or even text.
What makes generative AI unique is its ability to learn from uncertainty and explore the vast possibilities within data. By utilizing a two-part system consisting of a generator and a discriminator network, GANs create a feedback loop where they constantly improve each other’s performance. The generator attempts to produce content indistinguishable from real data while the discriminator learns to identify what is real versus what is generated. This dynamic between creation and critique enables GANs to generate novel and diverse outputs that are often astounding in their creativity.
By harnessing generative AI in your projects or personal explorations, you can unlock endless opportunities for innovation and creativity. From generating realistic artwork to developing virtual characters with unique personalities or even generating personalized music recommendations tailored specifically for each individual’s taste – there are countless applications for generative AI across various industries and domains.
Tip 1: Understand the basics of AI
Understanding the basics of AI is essential when starting with generative AI. Artificial Intelligence, or AI, is the technology that allows machines to perform tasks that normally require human intelligence. It involves machine learning algorithms, which enable computers to analyze data and make decisions based on patterns and trends. By familiarizing yourself with the key concepts of AI, such as neural networks and deep learning, you can gain a better understanding of how generative AI works.
Generative AI refers to algorithms that generate new content based on existing data. This could include anything from creating music compositions to generating realistic images or even writing original articles (yes, like this one!). By grasping the fundamentals of AI, you’ll be able to appreciate how these algorithms operate and why they can produce such impressive results. Moreover, being knowledgeable about the basics will enable you to effectively communicate your ideas and collaborate with others in this exciting field. So take the time to learn about AI’s principles; it will undoubtedly enhance your journey into generative AI!
Tip 2: Choose the right framework
When it comes to getting started with generative AI, one crucial factor to consider is choosing the right framework. With so many options available, it can be overwhelming to decide which one will best suit your needs. However, taking the time to research and explore different frameworks can make all the difference in your AI journey.
Firstly, it’s important to understand that each framework has its own strengths and weaknesses. Some frameworks excel in image generation, while others may be more suitable for natural language processing tasks. By understanding your specific goals and requirements, you can narrow down your choices and select a framework that aligns with your project objectives.
Furthermore, consider the community support and ease of use that each framework offers. A thriving community can provide valuable resources, tutorials, and forums where you can seek help when needed. Additionally, a user-friendly interface and good documentation are crucial for beginners who want to quickly grasp the fundamentals of generative AI. So before diving into any specific framework, take some time to assess how well it fits within your skill level and preferred learning style.
Overall, selecting the right framework is a pivotal step towards successful implementation of generative AI. With careful consideration of specific functionalities required for your project along with community support and ease of use aspects, you’ll find yourself on an exciting path filled with endless possibilities in generative AI development.
Tip 3: Gather and preprocess your data
Gathering and preprocessing your data is a critical step in getting started with generative AI. The quality and quantity of the data you have will directly impact the output and performance of your generative model. When gathering data, it’s important to ensure that it is diverse and representative of the real-world scenarios you want your model to generate. This can involve collecting a wide range of examples or using publicly available datasets.
Once you have gathered your data, preprocessing becomes crucial to ensure its suitability for training your model. Preprocessing involves tasks like cleaning, normalizing, and transforming the data to make it more manageable for machine learning algorithms. This step helps remove noise or outliers that could negatively impact the training process. It also allows you to merge multiple sources of data into a cohesive dataset.
Remember that preprocessing goes beyond just cleaning up the data; it can also involve augmenting or enriching it with additional information or features that might enhance the performance of your generative model. By carefully curating and prepping your dataset, you lay a strong foundation for generating high-quality outputs from your AI system.
Tip 4: Start with simple models
When diving into the world of generative AI, it’s easy to get swept away by the complexity of advanced models and techniques. However, it’s important not to overlook the power of starting with simple models. Simple models provide a solid foundation for understanding the basics of generative AI and allow you to grasp fundamental concepts before moving on to more complex architectures.
Starting with simple models also helps prevent you from feeling overwhelmed or discouraged by the complexity that can come with more advanced techniques. By building a strong understanding of basic principles, you can gradually scale up your knowledge and skills without getting lost in the intricacies of complicated models.
Moreover, simple models often have fewer hyperparameters to tune, making them easier and quicker to train. This means you can iterate faster and experiment with different ideas more efficiently. Additionally, working with simpler models allows for easier interpretation of results and facilitates debugging when something goes wrong.
In conclusion, starting with simple generative AI models is a smart strategy that enables you to build a strong foundation while avoiding many pitfalls associated with complexity and scalability. So don’t be afraid to take it slow at first – mastering simpler methods will ultimately empower you to tackle more complex challenges in the exciting world of generative AI.
Tip 5: Experiment and iterate
Experimentation and iteration are essential when working with generative AI. The field of generative AI is constantly evolving, and there is always room for improvement and innovation. By experimenting with different techniques, models, and approaches, you can uncover new possibilities that may not have been explored before.
One way to experiment is by tweaking the hyperparameters of your model. Hyperparameters control the behavior of the model during training, such as the learning rate or batch size. By systematically adjusting these values and observing how they affect the output, you can fine-tune your model to generate more accurate or creative results.
Moreover, iteration plays a crucial role in improving generative AI models. It’s rare to achieve perfect results on the first try, so it’s important to iterate and refine your approach over time. This could involve collecting feedback from users or domain experts, analyzing generated outputs for errors or biases, or even gathering additional data to train your model on. Each iteration brings you closer to achieving your desired outcome while also allowing you to learn from any mistakes made along the way.
In conclusion, experimentation and iteration are key ingredients for success in generative AI. By continuously refining your models through experimentation and embracing an iterative approach towards improvement, you can push the boundaries of what generative AI is capable of achieving. So don’t be afraid to try new ideas, explore different techniques, and adapt as you go – because true innovation comes from never settling for what’s already been done.
Conclusion: Embrace the possibilities of generative AI
In conclusion, it is clear that embracing the possibilities of generative AI can lead to exciting and transformative outcomes across various industries. By harnessing the power of artificial intelligence to generate new content, designs, or ideas, businesses can unlock a whole new realm of creativity and innovation. Whether it’s creating unique artwork, generating realistic human faces, or enabling virtual assistants with natural language processing capabilities, generative AI has the potential to revolutionize how we create and interact with technology.
However, it’s important to remember that while generative AI offers immense opportunities, ethical considerations should not be overlooked. As we continue to develop and refine AI models capable of generating content autonomously, questions around accountability and ownership arise. Who owns the generated creations? How do we ensure fairness in their distribution? These are complex issues that need careful thought and regulation.
Despite these challenges, there is no doubt that generative AI holds great promise for shaping the future of our digital landscape. By embracing its possibilities today and steering its development ethically tomorrow, we can pave the way for a world where creativity knows no bounds. So let’s embrace this incredible technology with open arms and see where it takes us – the possibilities are limitless.
